It’s no secret that performance reviews are flawed. Not only is occasional feedback unlikely to affect meaningful growth, but the feedback itself can also be suspect — studies indicate that numerical ratings reveal more about the rater than the person being reviewed, while open-ended evaluations are subject to a host of performance review biases.
Despite this research, most companies still rely on some form of performance review. They’re not ready to pivot from the idea of using reviews to promote professional development, and many employees aren’t either. As an engineering leader, you may not be able to overhaul your company’s review process, but you can still take steps to minimize some of its flaws. Engineering data found in your VCS and project management tools can help by acting as a check against anecdotal evidence and gut feel, helping you combat some common performance review biases.
Common Performance Review Biases
Biases may be evident in nearly every aspect of your day-to-day, but the open-ended format of most performance review frameworks is particularly vulnerable to some common biases. If you’re aware of their existence, you can take steps to counteract them.
Recency Bias
When reviews happen infrequently, the period of time right around review season is freshest in the reviewer’s mind and tends to be given the most weight.
What you can do: A skim of the Issues a developer worked on over the past year can be an important reminder of all they contributed, and a great way to refresh your memory. In addition, a deep dive into specific engineering metrics can help you distinguish longstanding patterns from recent anomalies. For example, you may have noticed that a developer is prioritizing their own work and putting off reviewing teammates’ Pull Requests. By looking at trends in a metric like Review Speed, you can determine whether or not that’s a new development, so you can calibrate your conversation accordingly.
Halo/Horns Effect
The Halo/Horns Effect occurs when a manager lets one trait — good or bad — skew their entire impression of an individual.
What you can do: There may be an engineer on your team who rarely speaks during meetings, and only participates when directly spoken to. You could take that as evidence that they’re generally disengaged at work, but data might reveal otherwise. If that same engineer has a consistently high PR Throughput and frequently participates in Code Review, it’s more likely that they just don’t like speaking up in group settings. With this information, you can offer specific feedback about their participation in meetings, rather than general (and inaccurate) feedback about their overall level of engagement, or you can adapt your expectations to match their work style.
Gender Bias
Studies show that reviewers tend to focus more on the personality traits of women and female-presenting individuals than on their skills or accomplishments.
What you can do: Make a conscious effort to focus on an individual’s work, and be sure to check any of your assumptions against objective data. For example, you can look at a developer’s history of commits, pushes, and review activity to confirm whether their work is in line with your expectations for a developer in their role. You might expect a more senior developer to spend less time committing new code and more time giving feedback to their teammates, but your expectations may be the opposite for a more junior team member.
Similarity Bias
This is the tendency of a manager to look more favorably on team members who remind them of themselves, perhaps due to a particular personality trait, a shared work style, or an aspect of their training or background.
What you can do: You may feel a particular kinship with a developer and assume that they’re achieving at the level you would in their role, but a look at the data — whether it’s their PR Throughput or a review of their contributions to Code Reviews — can help you regain perspective and ground your assessment in the reality of their work.
Using Data to Check Your Performance Review Biases
Data is not only a valuable tool for dismantling performance review biases, it can help you deliver specific, actionable feedback and collaborate with developers to set clear goals for the future. As with any tool, however, it must be used carefully.
Quantitative data should always be contextualized with qualitative data, and it’s important to resist the urge to compare or rank the members of your team. Developers coding new features will naturally work at a different pace than those working through technical debt, and team leads will likely be focused more on coaching or project management than committing code. You’ll need to pair data with an understanding of the circumstances surrounding each team member’s work in order to have a complete picture of their overall performance.
If you’re interested in learning more about incorporating data into your performance reviews, speak to a Velocity product specialist.
Get articles like this in your inbox.
Trending from Code Climate
1.
Engineering Leaders Share Thoughts on Leadership in Disrupted Times in a New Survey
For engineering teams, disruption to the business can have a significant impact on the ability to deliver and meet goals. These disruptions are often a result of reprioritization and budget changes on an organizational level, and are amplified during times of transition or economic instability.
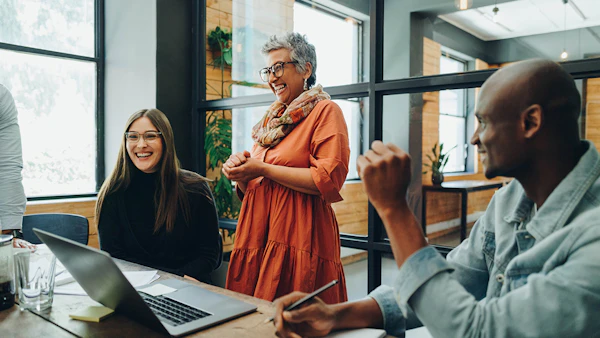
2.
Built In’s 2023 Best Places to Work — Why Code Climate Made the List
At Code Climate, we value collaboration and growth, and strive for greatness within our product and workplace. For us, this means fostering a supportive, challenging, people-first culture. Thanks to an emphasis on these values, we’ve earned spots on three of Built In’s 2023 Best Places to Work awards lists, including New York City Best Startups to Work For, New York City Best Places to Work, and U.S. Best Startups to Work For.
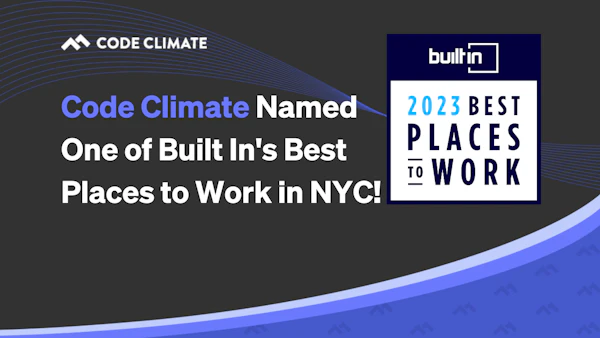
3.
Turnkey Deployment Delivers Day-One Value for Yottaa
Learn how Yottaa gained immediate value from Code Climate Velocity right out of the box.
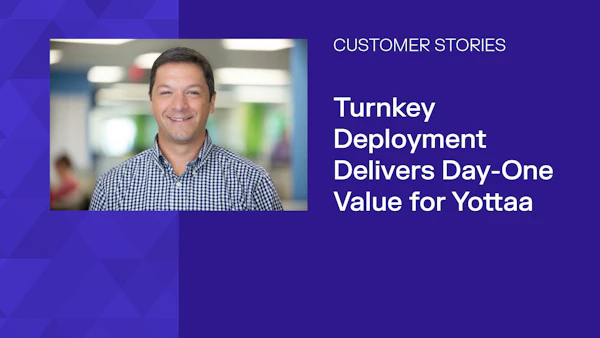
Get articles like this in your inbox.
Get more articles just like these delivered straight to your inbox
Stay up to date on the latest insights for data-driven engineering leaders.