By 2025, McKinsey anticipates that the majority of enterprise employees will leverage data to improve decision-making and automate activities. This doesn’t come as a surprise to folks who have witnessed first-hand how important data is for modern enterprises. Engineering data, in particular, provides valuable insights into team performance and enables businesses to make informed decisions to improve the flow of work.
While many companies will use data in the next year, not all will be at the same stage in their journey — some will have just begun looking at their organization’s data, while others will already have a culture of analytical rigor and data-driven decision-making in place. As businesses mature in their data journey, what they measure and how they act on it evolves; it takes time to get comfortable working with data.
At Code Climate, we’ve had the honor of guiding leading enterprises on this journey and have identified five common stages organizations go through, as well as key milestones and goals that characterize each stage. Most companies begin by using data to inform basic decisions before they are able to leverage it to systematically improve predictability, team health, and efficiency. The organizations that are most mature in their use of data continue to find new ways to prioritize learning, embrace measurement, and celebrate successes, even when they appear to have achieved them. Here’s a look at the five phases of the data journey and how engineering leaders can use metrics to improve productivity and performance at each stage.
Stage 1: Introduce data-driven processes and decision-making
Introducing data-driven processes and decision-making is the first step in instilling analytical rigor and replacing gut feelings with objective data. In this foundational stage, organizations begin to set, measure, and automate reporting for team goals and objectives and key results (OKRs). Establishing goals and measuring progress against them is key to driving a culture of continuous improvement.
Organizations beginning to utilize data will need to start the work of mapping engineering outcomes back to business objectives, and choosing relevant goals. For example, if an organization is looking to reduce disruptions due to incidents and bugs, engineering may want to focus on improving a metric like Change Failure Rate, which measures the percentage of deployments causing failures in production. If there’s a critical feature that engineering is working to get to market, it may be more important to look at something like Cycle Time, which serves as a measure of engineering speed. At this stage, some enterprises also combine engineering and business metrics in a business intelligence (BI) tool to get a comprehensive view of the organization's performance.
As long as business objectives remain top-of-mind, it can also be instructive to look at big-picture measures of speed, output, and quality across teams. This can help highlight areas for improvement and reveal best practices that can be useful if adopted throughout the organization.
In order to access their data and attendant insights, many organizations leverage a Software Engineering Intelligence (SEI) platform like Code Climate Velocity, which automatically cleans, links, and normalizes data gleaned from the tools engineers are already using. An SEI platform provides visibility into engineering practices to help leaders boost productivity and efficiency, improve team health, and maximize engineering impact. Velocity integrates with common BI tools via an API to facilitate seamless data collection and analysis.
Stage 2: Gain critical visibility and predictability
Once organizations have introduced data-driven processes, they can leverage insights from engineering data to identify areas in need of improvement, like flawed code review processes or poor communication. Having visibility into what teams and individuals are working on also provides insights into workload distribution and potential bottlenecks. It helps teams prioritize tasks, make informed decisions, and align efforts with organizational goals. Proactively revealing unplanned engineering work helps manage and allocate resources to minimize disruptions and delays.
At this stage, having standardized measures enables unification of reporting across business units, making it possible to get a holistic view of engineering performance and facilitate data-driven decision-making at both the team and organizational levels. It also helps engineering organizations understand performance across teams to identify top performers and areas for improvement. For example, metrics like PR Throughput, Cycle Time, and Time to First Review help large organizations, like Faire, identify changes in team performance that might signal issues to be addressed. Faire has leveraged these insights to help teams hit record output levels per person, increasing PR Throughput by 60% and Push Count by 65% in a single year, all while nearly quadrupling the size of their engineering team.
Stage 3: Use engineering metrics to optimize team health
Once a team has created a productive culture around data and understands what data can reveal about its organization, it can then take on the more nuanced work of using data to improve team health. It's not until developers feel safe with metrics and understand how they can be used that this will be done well, but once an organization is ready, data can be a critical tool for coaching, setting goals, and instituting best practices.
At this stage, engineering managers can use metrics to better coach their teams and identify areas for improvement. Analyzing engineering metrics will reveal top-performing teams and individuals, allowing leaders to set best practices, share knowledge, and provide support where needed. Standardizing code reviews based on data helps maintain code quality and proactively identify potential roadblocks or areas of improvement. Additionally, engineering metrics enable organizations to measure new hires’ contributions and ramp time to assess their progress and integration into the team.
Stage 4: Improve the SDLC with software engineering efficiency metrics
Even the highest-performing organizations battle through bottlenecks and blockers to deliver quality code. Engineering leaders need visibility to identify recurrent slowdowns and inefficiencies so they can improve processes and help their teams become more efficient.
When developers see how data can help them improve, they can then work together to better team processes as well. Tracking key value stream metrics and other data points makes it possible for teams to accelerate software delivery by identifying areas of friction and working together to resolve them. Using an SEI platform provides a centralized view of metrics across the SDLC, enabling teams to gain insights and make informed decisions at each stage. Analyzing the different stages of the engineering process helps identify opportunities to reduce excess work and increase efficiency. For example, when the commercial real estate technology company, VTS, set Cycle Time as a north star metric, engineering teams could then isolate key segments of the development process with their corresponding metrics, for example, measuring Review Speed or Review Cycles as a way to gain deeper insight into the Code Review Process. They were also able to reinforce efficiency-focused best practices, like keeping PR Sizes small. Ultimately, they were able to boost their speed 30%, while also doubling their deployment frequency.
Using an SEI platform helps leaders ingest, link, and clean data from the engineering tools their teams are already using, so they can view metrics from across the SDLC. Doing this allows them to monitor the four DORA metrics — Deployment Frequency, Mean Lead Time for Changes, Mean Time to Recover, and Change Failure Rate — and other engineering metrics to benchmark to industry standards for software delivery and DevOps maturity.
Stage 5: Empower teams to build a culture of excellence
Top-performing organizations prioritize learning, embrace measurement, and celebrate successes so they can continue to excel. With coaching and process improvements in place, organizations will benefit from formalizing their project of continuous improvement. The previous four stages of the engineering data journey culminate in achieving this level of maturity.
At this stage, organizations often create a role or team dedicated to engineering excellence and enablement. This is often the phase when teams can best leverage frameworks to help them achieve continuous improvement, collaboration, and high performance.
Empowering teams to build a culture of excellence requires that managers have the tools to measure and improve their teams. With an SEI platform, engineering leaders can enhance planning accuracy, benchmark performance against top-performing organizations, embrace management frameworks like DORA and effectively communicate successes and impact to stakeholders. By implementing these practices, organizations foster a culture of continuous learning, growth, and excellence in their engineering teams, which leads to shipping more — and better — products.
To find out more about how an SEI platform can support you in your data journey, speak to a Velocity specialist.
Trending from Code Climate
1.
How to Navigate New Technology Expectations in Software Engineering Leadership
Rapid advancements in AI, No-Code/Low-Code, and SEI platforms are outpaced only by the evolving expectations they face. Learn how engineering leaders can take actionable steps to address new technology challenges.
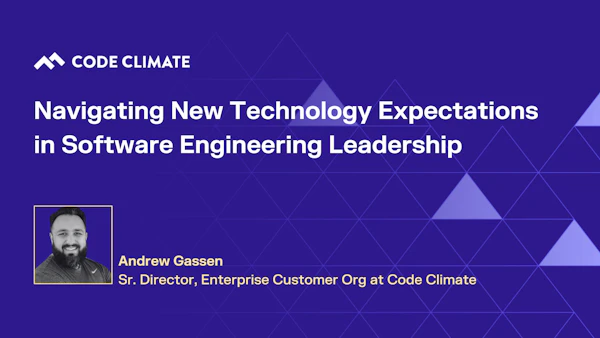
2.
Mapping Engineering Goals to Business Outcomes
Understanding how engineering activities impact business objectives enables engineering leaders to make informed strategic decisions, keep teams aligned, advocate for resources, or communicate successes.
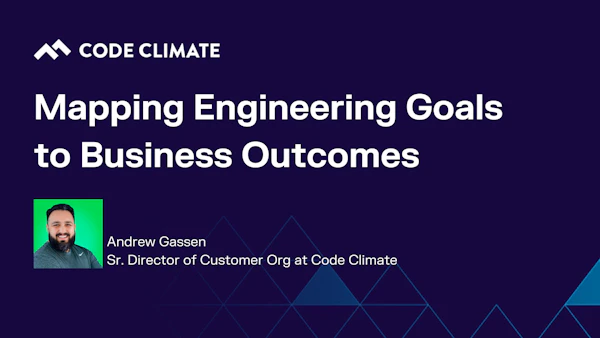
3.
Unlocking Efficiency: Optimizing Pull Request Reviews for Enterprise Engineering Teams
As engineering teams grow, so can the complexity of the code review process. From understanding industry benchmarks to improving alignment across teams, this article outlines strategies that large engineering organizations can use to optimize Review Cycles.
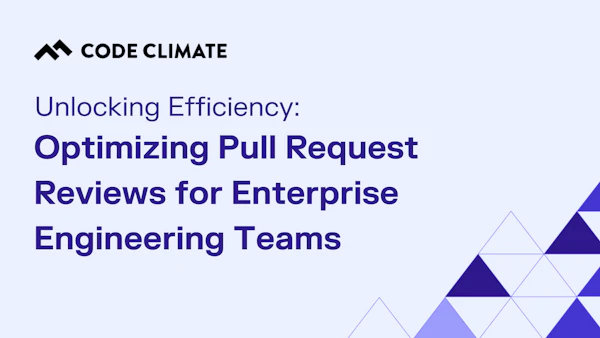
Get articles like this in your inbox.
Get more articles just like these delivered straight to your inbox
Stay up to date on the latest insights for data-driven engineering leaders.